Studying at the University of Verona
Here you can find information on the organisational aspects of the Programme, lecture timetables, learning activities and useful contact details for your time at the University, from enrolment to graduation.
Academic calendar
The academic calendar shows the deadlines and scheduled events that are relevant to students, teaching and technical-administrative staff of the University. Public holidays and University closures are also indicated. The academic year normally begins on 1 October each year and ends on 30 September of the following year.
Course calendar
The Academic Calendar sets out the degree programme lecture and exam timetables, as well as the relevant university closure dates..
Period | From | To |
---|---|---|
I semestre | Oct 1, 2020 | Jan 29, 2021 |
II semestre | Mar 1, 2021 | Jun 11, 2021 |
Session | From | To |
---|---|---|
Sessione invernale d'esame | Feb 1, 2021 | Feb 26, 2021 |
Sessione estiva d'esame | Jun 14, 2021 | Jul 30, 2021 |
Sessione autunnale d'esame | Sep 1, 2021 | Sep 30, 2021 |
Period | From | To |
---|---|---|
Festa dell'Immacolata | Dec 8, 2020 | Dec 8, 2020 |
Vacanze Natalizie | Dec 24, 2020 | Jan 3, 2021 |
Vacanze Pasquali | Apr 2, 2021 | Apr 5, 2021 |
Festa del Santo Patrono | May 21, 2021 | May 21, 2021 |
Festa della Repubblica | Jun 2, 2021 | Jun 2, 2021 |
Vacanze estive | Aug 9, 2021 | Aug 15, 2021 |
Exam calendar
Exam dates and rounds are managed by the relevant Science and Engineering Teaching and Student Services Unit.
To view all the exam sessions available, please use the Exam dashboard on ESSE3.
If you forgot your login details or have problems logging in, please contact the relevant IT HelpDesk, or check the login details recovery web page.
Academic staff
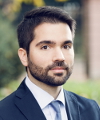
Bullini Orlandi Ludovico
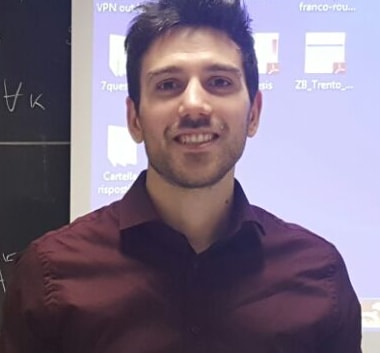
Cordoni Francesco Giuseppe
Study Plan
The Study Plan includes all modules, teaching and learning activities that each student will need to undertake during their time at the University.
Please select your Study Plan based on your enrollment year.
1° Year
Modules | Credits | TAF | SSD |
---|
2° Year activated in the A.Y. 2021/2022
Modules | Credits | TAF | SSD |
---|
Modules | Credits | TAF | SSD |
---|
Modules | Credits | TAF | SSD |
---|
Modules | Credits | TAF | SSD |
---|
1 module among the following (1st year: Big Data epistemology and Social research; 2nd year: Cybercrime, Data protection in business organizations, Comparative and Transnational Law & Technology)
2 courses among the following (1st year: Business analytics, Digital Marketing and market research; 2nd year: Logistics, Operations & Supply Chain, Digital transformation and IT change, Statistical methods for Business intelligence)
2 courses among the following (1st year: Complex systems and social physics, Discrete Optimization and Decision Making, 2nd year: Statistical models for Data Science, Continuous Optimization for Data Science, Network science and econophysics, Marketing research for agrifood and natural resources)
2 courses among the following (1st year: Data Visualisation, Data Security & Privacy, Statistical learning, Mining Massive Dataset, 2nd year: Machine Learning for Data Science)
Legend | Type of training activity (TTA)
TAF (Type of Educational Activity) All courses and activities are classified into different types of educational activities, indicated by a letter.
Statistical learning (2020/2021)
Teaching code
4S009067
Credits
6
Language
English
Scientific Disciplinary Sector (SSD)
INF/01 - INFORMATICS
The teaching is organized as follows:
Teoria
Credits
5
Period
II semestre
Academic staff
Laboratorio
Learning outcomes
The course aims to introduce students to the statistical models used in data science. The foundations of statistical learning (supervised and unsupervised) will be developed by placing the emphasis on the mathematical basis of the different state-of-the-art methodologies. It also aims to provide rigorous derivations of the methods currently used in industrial and scientific applications to allow students to understand their requirements for correct use. Laboratory sessions will illustrate the use of fundamental algorithms and industrial case studies in which the student will be able to learn to analyze real datasets by means of Python software.
At the end of the course the student has to show to have acquired the following skills:
● knowledge of the main stages of: data analysis and preparation
● ability to use the main regression models
● ability to develop pro-feature selection solutions
● ability to use regularization methods, e.g., ridge regression, LASSO, elastic net, least angle regression, and classification
● knowledge of unsupervised methods
● know and know how to develop algorithms in the field of dimensionality reduction, analysis of the main components (PCA), K-means clustering, hierarchical clustering, and cross-validation
Program
-- Linear models for Regression (Linear Regression, Subset Variable Selection, Shrinkage/Regularization)
-- Classification models (Logistic Regression, Linear Discriminant Analysis)
-- Tree Based Methods (Decision Trees, Bagging, Random Forest, Boosting)
-- Unsupervised methods (Principal Component Analysis, K-Means Clustering, Hierarchical Clustering)
-- Model Assessment and selection (cross validation)
-- Introduction to Neural Networks (Single layer neural network, training a neural network)
Lab:
-- Linear regression and related variable selection/shrinkage methods (in Python)
-- Classification with logistic regression (in Python)
-- Clustering with k-means and hierarchical clustering (in Python)
-- Artificial Neural Networks (in Python)
Examination Methods
The exam is composed of an oral test and the realization of a project that focuses on the application of statistical learning approaches to a specific case study.
Type D and Type F activities
Documents and news
-
Regolamento didattico 2020/2021 (pdf, it, 470 KB, 12/04/21)
years | Modules | TAF | Teacher |
---|---|---|---|
1° | The course provides an introduction to blockchain technology. It focuses on the technology behind Bitcoin, Ethereum, Tendermint and Hotmoka. | D |
Nicola Fausto Spoto
(Coordinator)
|
Career prospects
Module/Programme news
News for students
There you will find information, resources and services useful during your time at the University (Student’s exam record, your study plan on ESSE3, Distance Learning courses, university email account, office forms, administrative procedures, etc.). You can log into MyUnivr with your GIA login details: only in this way will you be able to receive notification of all the notices from your teachers and your secretariat via email and soon also via the Univr app.
Graduation
Deadlines and administrative fulfilments
For deadlines, administrative fulfilments and notices on graduation sessions, please refer to the Graduation Sessions - Science and Engineering service.
Need to activate a thesis internship
For thesis-related internships, it is not always necessary to activate an internship through the Internship Office. For further information, please consult the dedicated document, which can be found in the 'Documents' section of the Internships and work orientation - Science e Engineering service.
Final examination regulations
Upon completion of the Degree programme, students will need to submit and present their thesis/dissertation, which must be in English and focusing on a scientific topic covered during the programme. Alternatively, the thesis/dissertation may consist of the analysis and solution of a case study (theoretical and/or relevant to a real industrial context), experimental work, possibly developed as part of an internship, or original and independent research work that may include mathematical formalisation, computer design and a business-oriented approach.
These activities will be carried out under the guidance of a Thesis Supervisor at a University facility, or even outside the University of Verona, either in Italy or abroad, provided that they are recognised and accepted for this purpose in accordance with the teaching regulations of the Master's Degree programme in Data Science.
22 CFU credits shall be awarded for the final examination (assessment of the thesis/dissertation).
The Graduation Committee, which is in charge of the evaluation of the final examination (presentation of the dissertation in English) shall evaluate each candidate, based on their achievements throughout the entire degree programme, carefully assessing the degree of consistency between educational and professional objectives, as well as their ability for independent intellectual elaboration, critical thinking, communication skills and general cultural maturity, in relation to the objectives of the Master's Degree programme in Data Science, and in particular, in relation to the topics dealt with by the candidate in their thesis.
Students may take the final exam only after they have passed all the other modules and exams that are part of their individual study plan, and fulfil all the necessary administrative requirements, in accordance with the terms indicated in the General Study Manifesto.
The graduation exam and ceremony will be carried out by the Graduation Committee appointed by the Chair of the Teaching Committee and composed of a President and at least four other members chosen among the University's lecturers.
The thesis/dissertation will be assessed by the Dissertation Committee, which is composed of three lecturers possibly including the Thesis Supervisor, and appointed by the Chair of the Teaching Committee. The Dissertation Committee shall produce an evaluation of the dissertation, which will be submitted to the Graduation Committee, which will issue the final graduation mark. The Teaching Committee shall govern the procedures of the Dissertation Committee and the Graduation Committee, and any procedures relating to the score awarded for the final exam through specific regulations issued by the Teaching Committee.
Documents
Title | Info File |
---|---|
![]() |
pdf, it, 387 KB, 27/04/22 |
List of theses and work experience proposals
theses proposals | Research area |
---|---|
Domain Adaptation | Computer Science and Informatics: Informatics and information systems, computer science, scientific computing, intelligent systems - Computer graphics, computer vision, multi media, computer games |
Domain Adaptation | Computer Science and Informatics: Informatics and information systems, computer science, scientific computing, intelligent systems - Machine learning, statistical data processing and applications using signal processing (e.g. speech, image, video) |
Domain Adaptation | Computing Methodologies - IMAGE PROCESSING AND COMPUTER VISION |
Domain Adaptation | Computing methodologies - Machine learning |
Attendance
As stated in the Teaching Regulations for the A.Y. 2022/2023, attendance at the course of study is not mandatory.